Product Twin, AI Agents and Action Cloud in an intelligent low-code environment
Our Product Twin represents how a system works. Together with our underlying Knowledge Graph, it connects all product data and puts it into logical context. This makes it easy for engineers across all domains to consider critical dependencies in their flow of work supported by intuitive solutions with an agentic layer.
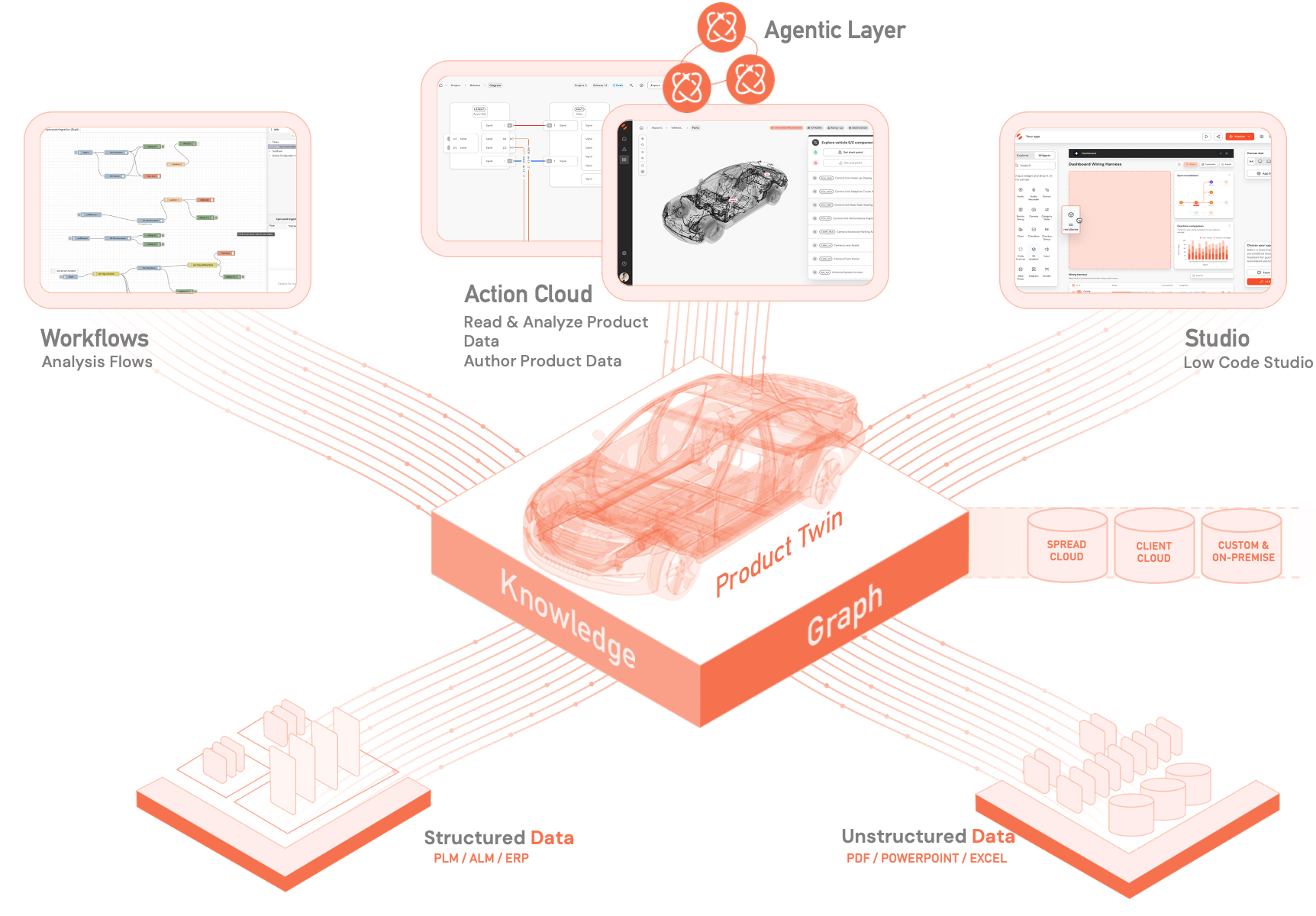
10x
faster time-to-market
50%
costs reduction
Superior
quality
How our customers benefit from SPREAD
How a global OEM sets up its source-of-engineering-truth
Smart connectors integrate product data into intuitive dashboards, giving inline reworkers real-time access to product descriptions, 3D wiring geometries, DTC mappings, and fault hotspots for proactive issue detection.
faster error correction
reduced process steps
reduced error searching time
How a global OEM sets up its source-of-engineering-truth
Connected systems integrate product data to drive specialized apps for project management and decision-making, offering dashboards with real-time status, readiness, and risk insights while proactively addressing cross-domain gaps and interdependencies.
faster to market
costs reduction
quality
How a global OEM enables a source-of-truth for product data
Integrated product data is connected via smart connectors, enabling workshops to access up-to-date repair guidance and wiring information while creating active feedback loops that enhance product data for improved field monitoring and innovation.
faster troubleshooting
lower warranty cost
increase in CSAT
4 steps to an end-to-end product data journey
From distributed product data to actionable Agentic Engineering Intelligence.
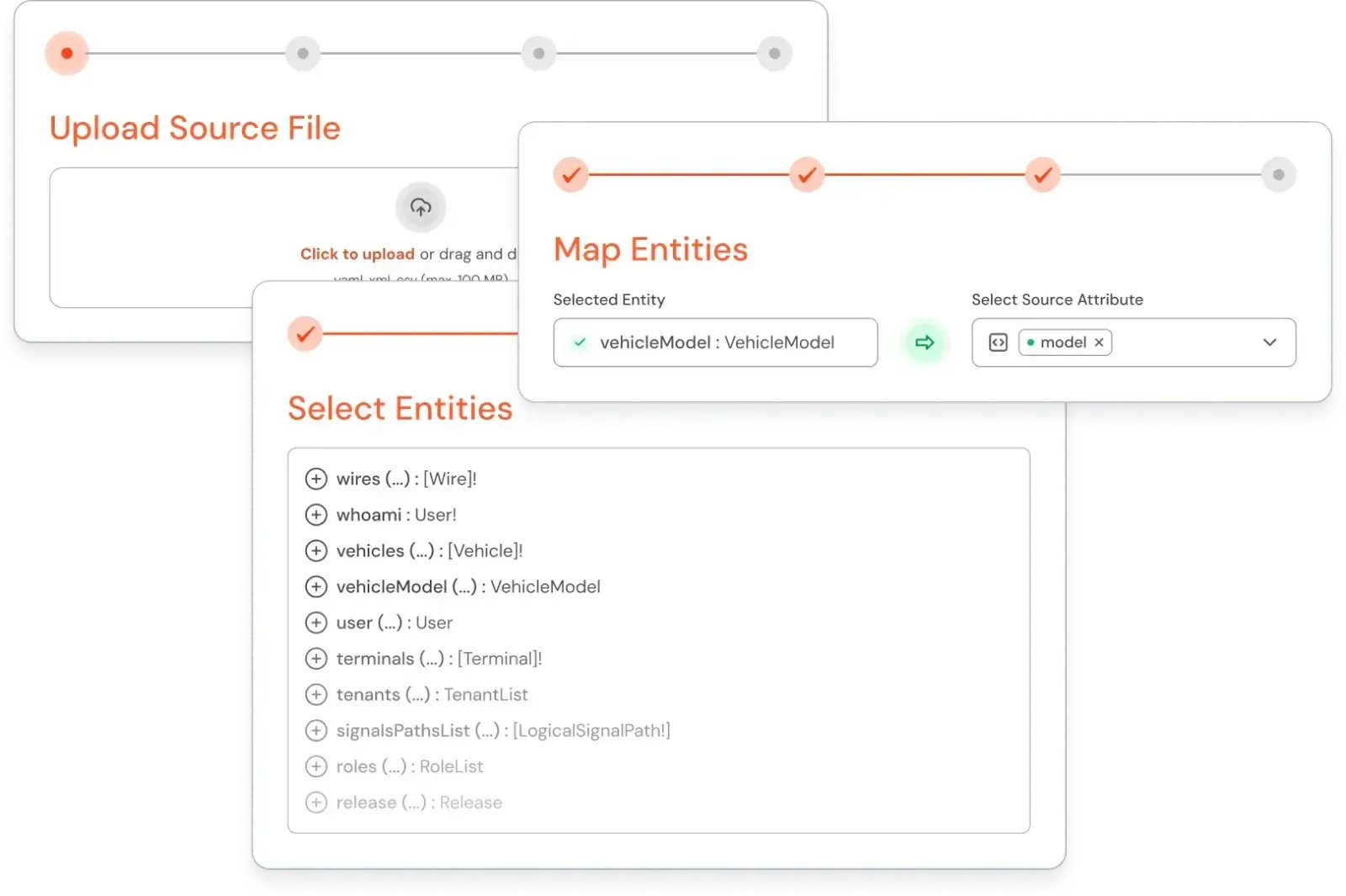
Connect data from various sources
Create a standardized interpretation of your data by integrating your different data sources, including existing systems, structured, or unstructured data.
Map data into context
Create logical connections by mapping the data to SPREAD’s normalized information model. This helps you quickly establish a comprehensive understanding of the data across domains.
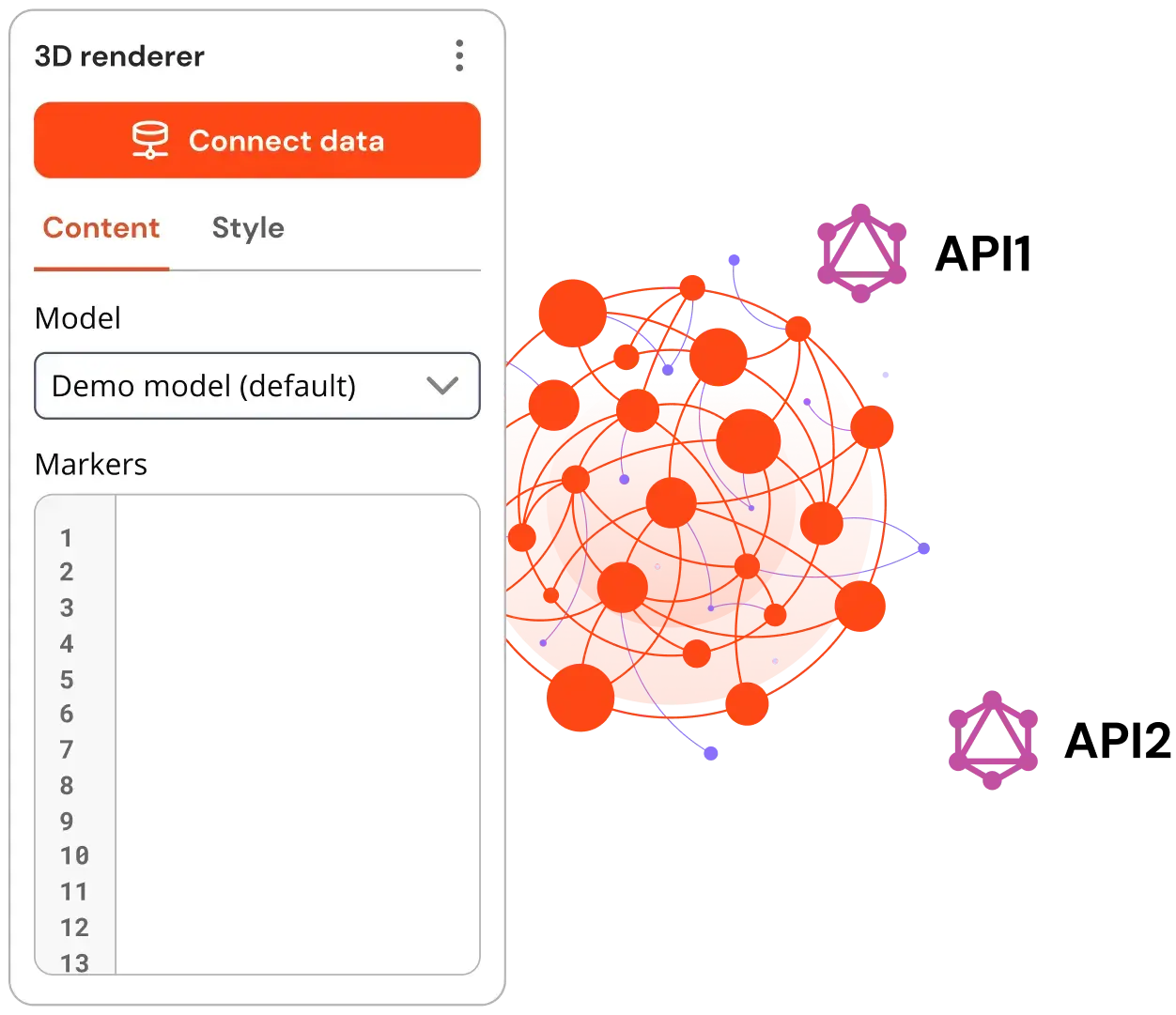
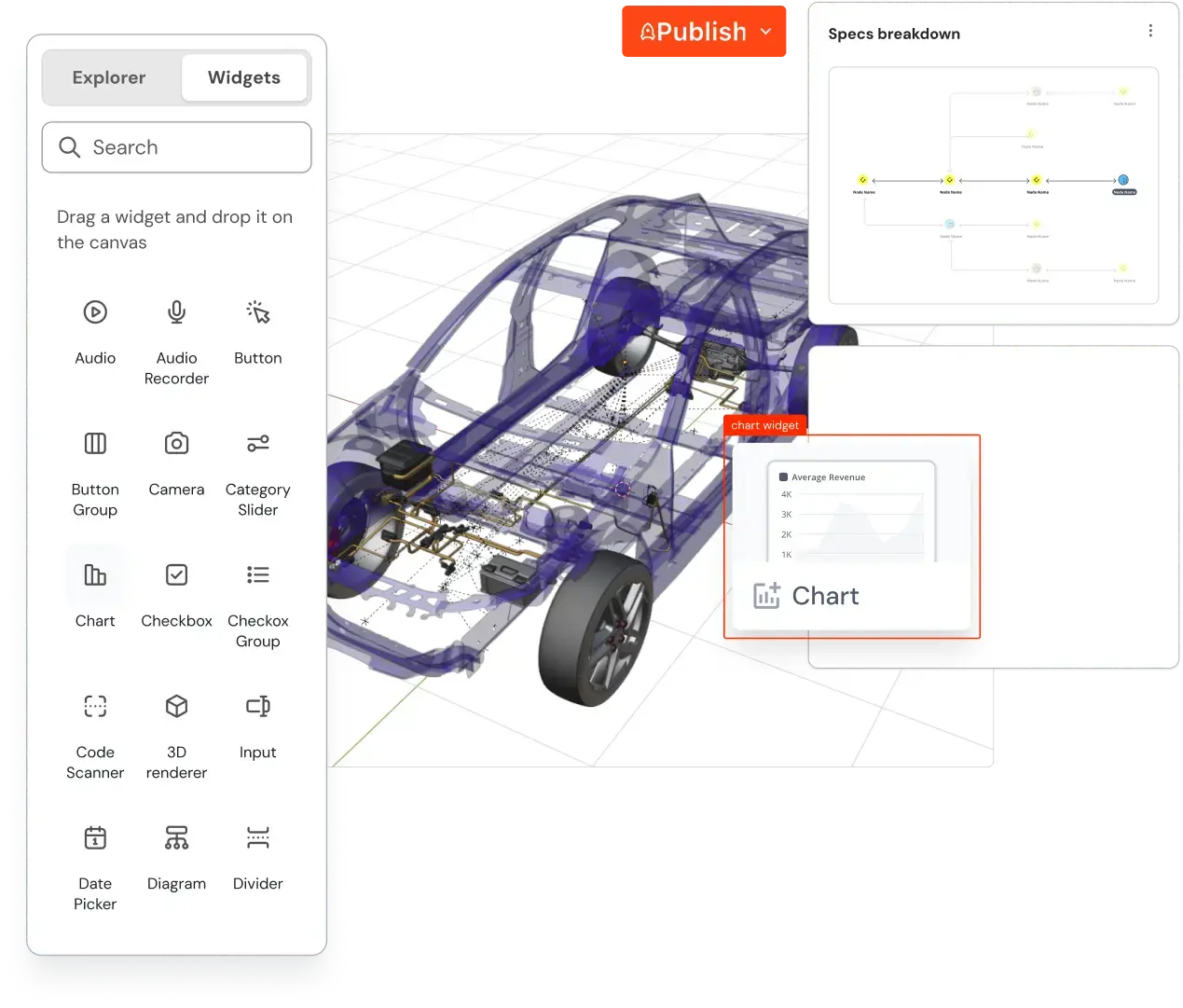
Use and customize our out-of-the box solutions
Use preconfigured applications or build custom ones that give you the control and flexibility you need over the product information required for your specific use case - with our low-code collaboration environment, AI Agents that allow you to interact in natural language and automate engineering tasks.
Get fast value from Agentic Engineering Intelligence
With data in context, you can simply select your solution and apply the insights where you need them – in R&D, Production, and Aftermarket.
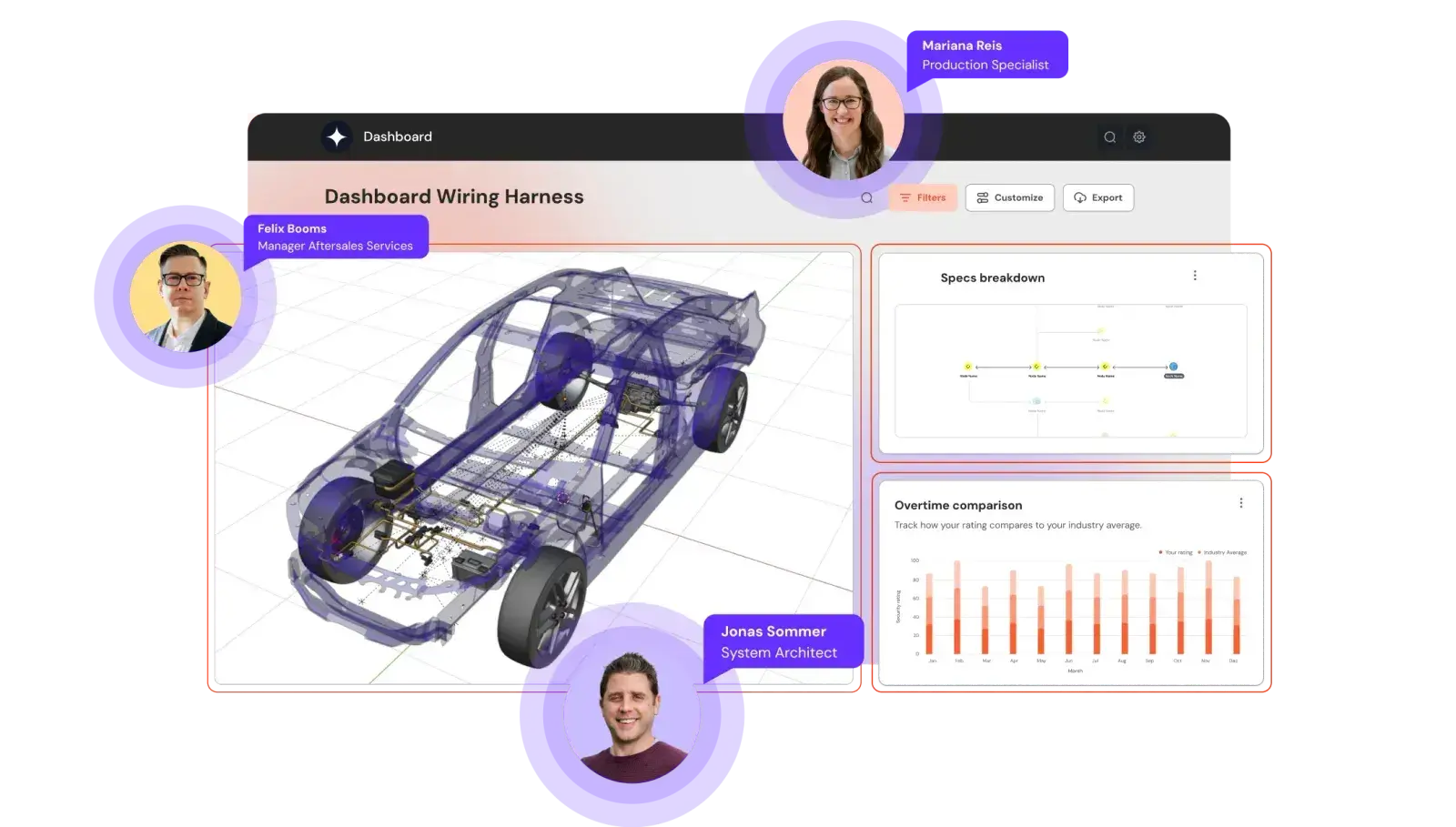
FAQs
SPREAD’s Agentic Engineering Intelligence is an AI-driven, federated system designed for engineering teams. It consists of three core layers:
-
Rapid Data Ingestion – Smart connectors integrate structured and unstructured data from CAD, PLM, ERP, IoT, and other sources into the Engineering Intelligence Graph.
-
Product Twin (PT) – A federated Knowledge Graph that contextualizes data across the product lifecycle, providing engineers with a unified, queryable data model.
-
Action Cloud – AI-driven automation and analytics enable engineers to conduct compliance checks, optimize requirements, and analyze dependencies efficiently.
This architecture transforms raw engineering data into context-aware, AI-actionable knowledge, reducing manual effort and improving decision-making.
SPREAD’s Engineering Intelligence Graph (EI Graph) is a federated supergraph that unifies structured and unstructured product data across domains (R&D, Production, Aftermarket).
It leverages:
-
GraphQL-based schema to standardize data models.
-
Subgraphs optimized per domain, aggregated into a unified, scalable supergraph.
-
Automated AI-assisted data mapping to integrate diverse engineering data sources.
This enables cross-domain insights, real-time querying, and advanced analytics, making complex product data machine-readable and actionable. If you like to learn more about it, please watch this video: https://www.spread.ai/resources/knowledge-hub/how-we-use-a-graphql-schema-as-a-contract-to-efficiently-build-applications
Yes. SPREAD provides out-of-the-box connectors, API integrations, and graph federation to ingest data from CAD, PLM, ERP, and IoT systems. Features include:
-
Declarative data mapping – Standardizing heterogeneous data formats.
-
Real-time synchronization – Ensuring up-to-date insights.
-
Federated query capabilities – Allowing seamless interaction with external data sources.
SPREAD enhances existing tool chains by unifying, structuring, and contextualizing data, eliminating silos.
SPREAD integrates and analyzes diverse product data across the entire lifecycle. This includes CAD design data, engineering specifications, production metrics, test results, maintenance logs, and real-world usage feedback.
By unifying structured and unstructured data from multiple sources, SPREAD creates a comprehensive, interconnected view that enables engineers to understand complex dependencies, make data-driven decisions, and optimize product development and support processes.
EI Agents are AI-driven routines operating within SPREAD’s Engineering Intelligence Graph. They can be taught to automate engineering tasks by interpreting queries, accessing structured and unstructured data, and providing validated, context-aware insights.
These agents streamline workflows, enhance data mapping, and support high-value tasks like requirements analysis, error pattern detection, and specification generation.